Generative AI vs. Traditional AI: A Comparative Analysis
- WriteMe. ai
- Nov 1, 2023
- 4 min read
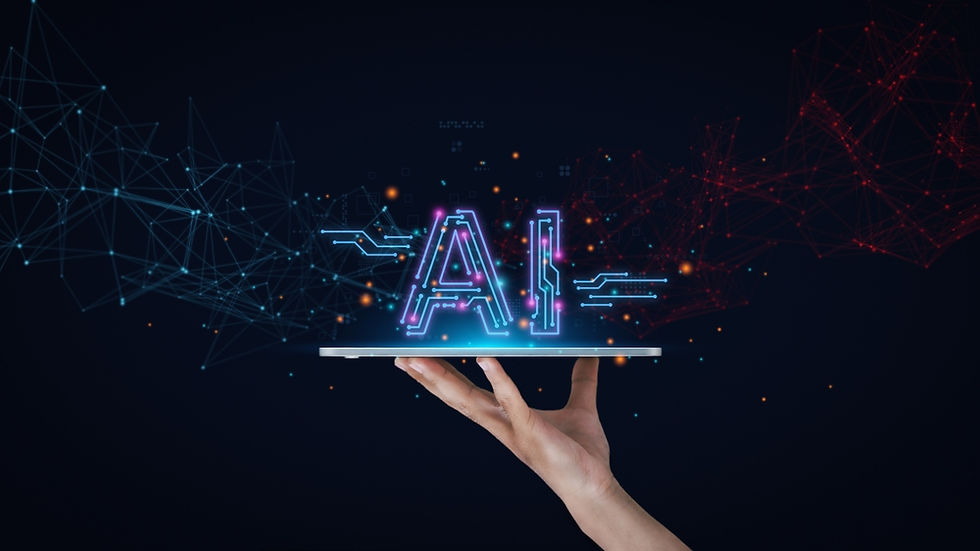
Artificial Intelligence (AI) has rapidly evolved in recent years, with one of the most significant advancements being the emergence of generative AI. Traditional AI and generative AI represent two distinct approaches to solving problems, automating tasks, and creating intelligent systems. In this article, we will explore the key differences between generative AI and traditional AI, their applications, and the implications for various industries.
Understanding Traditional AI
Traditional AI, often referred to as rule-based or expert systems, relies on explicit programming and predefined rules to make decisions and perform tasks. It involves writing code that dictates the system's behavior, and the AI writer based on these predetermined instructions. This approach has been the foundation of AI for many years and is effective in solving specific, well-defined problems.
Key Characteristics of Traditional AI:
Explicit Rules: Traditional AI systems follow strict, predefined rules, making them well-suited for tasks with clear guidelines and outcomes.
Narrow Focus: These systems are typically specialized for a particular task or domain, and they lack the flexibility to adapt to new, unforeseen challenges.
Structured Data: Traditional AI primarily operates on structured data, such as databases and spreadsheets, where the rules can be precisely defined.
Supervised Learning: Supervised learning is a common method in traditional AI, where models are trained on labeled datasets.
The Rise of Generative AI
Generative AI, on the other hand, represents a departure from the rule-based approach. It harnesses the power of neural networks, particularly deep learning models like GANs (Generative Adversarial Networks) and transformers, to create AI systems that can generate content and make decisions based on patterns and data rather than explicit rules. Generative AI has gained prominence due to its ability to handle unstructured data and produce creative outputs.
Key Characteristics of Generative AI:
Data-Driven: Generative AI relies on large datasets to learn and generate content. It is capable of understanding patterns and context within data.
Creativity: These systems can produce novel content, whether it's in the form of text, images, music, or other media.
Unstructured Data: Generative AI excels in handling unstructured data, such as natural language text, images, and audio.
Self-Improvement: Generative AI models can improve over time as they receive more data, making them adaptable to changing circumstances.
Applications of Traditional AI
Traditional AI has found success in various applications, including:
Expert Systems: Traditional AI has been used to develop expert systems in fields like medicine, where it can diagnose diseases based on predefined rules and medical knowledge.
Manufacturing and Robotics: In manufacturing, traditional AI controls robotic arms to perform precise tasks like assembly and welding.
Recommendation Systems: Traditional AI powers recommendation engines, which suggest products, movies, or music based on user behavior and preferences.
Search Engines: Search algorithms, used by search engines like Google, employ traditional AI to rank and retrieve web pages based on specific criteria.
Financial Analysis: Traditional AI is used for algorithmic trading and fraud detection in the financial industry.
The Diverse Landscape of Generative AI
Generative AI, with its ability to generate creative content and understand context, has opened up new possibilities in various domains:
Natural Language Processing (NLP): Models like GPT (Generative Pre-trained Transformer) have revolutionized NLP, enabling chatbots, automated content generation, and more.
Image Generation: Generative AI, particularly GANs, is used to create lifelike images and videos, including deepfake technology.
Art and Creativity: Generative AI has been employed in art, music, and creative writing, producing original works based on patterns in existing content.
Healthcare: In healthcare, generative AI assists in medical image analysis, drug discovery, and generating patient reports.
Autonomous Vehicles: Generative AI plays a role in the development of self-driving cars, helping them make real-time decisions based on sensor data.
Comparing Performance and Flexibility
One significant distinction between generative AI and traditional AI lies in their performance and flexibility. Generative AI excels in tasks that require understanding context, creativity, and adaptation to unstructured data. It can surpass traditional AI in areas like natural language understanding, content generation, and image recognition. Traditional AI, while rigid in comparison, remains highly effective for tasks where rules and structure are paramount. For example, manufacturing processes that demand precision, or financial calculations that rely on fixed algorithms, continue to benefit from traditional AI.
Real-World Examples
Let's delve deeper into a couple of real-world examples to illustrate the differences and applications of generative AI and traditional AI. 1. Chatbots: Generative AI: Chatbots powered by generative AI, such as OpenAI's GPT-3, are capable of engaging in more natural and context-aware conversations. They can understand and respond to user queries with a human-like touch. Traditional AI: Rule-based chatbots operate on predefined decision trees and scripted responses. They lack the ability to grasp the nuances of language and context in the same way that generative AI models can. 2. Healthcare: Generative AI: In medical image analysis, generative AI can identify and generate insights from complex images like MRIs and X-rays. It can help in early disease detection and personalized treatment recommendations.
The Future and Ethical Considerations
Both generative AI and traditional AI have their places in the AI landscape. The future likely involves a hybrid approach where these two paradigms complement each other. However, as AI becomes increasingly sophisticated, ethical considerations become paramount. Generative AI, in particular, raises concerns about deepfakes, misinformation, and privacy violations. It's essential for developers and policymakers to establish guidelines and regulations to ensure responsible AI development and usage.
Conclusion
the choice between generative AI and traditional AI depends on the specific task at hand. Understanding the strengths and limitations of each approach is vital for selecting the right AI solution for a particular problem. The dynamic AI landscape continues to evolve, and embracing both generative and traditional AI can lead to innovative solutions across various industries. It's an exciting time in the field of artificial intelligence, and the synergy between these two approaches promises a bright future for AI applications.
Comments